A New Framework
Dr. Ken Seiden is a managing director with Navigant, where he heads the Energy practice’s Data Science team. Over the last thirty years, he has assisted dozens of utilities in developing more accurate peak demand and energy sales forecasts.
Brian Eakin is an associate director at Navigant, advising utilities on developing and operationalizing a data analytics strategy, identifying actionable insights, and prioritizing opportunities for strategic improvement.
Load forecasting errors have grown to an unprecedented level, and the corresponding risks are astounding. Flat or declining loads, downward pressure on earnings, customer-owned distributed generation, energy efficiency programs, and new codes and standards are the new normal. Casting blame on the weather is a common refrain.
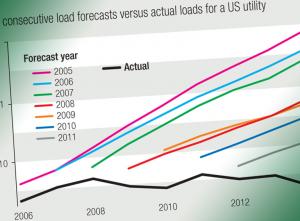
The time has come for a more comprehensive approach to load forecasting that can address the increasing complexities of the Energy Cloud. If you are a utility executive concerned about suffering significant forecast misses and want guidance on how to fix the problem, keep reading.
See Figure One.
The focus of this article is on long-term forecasting, which we define as anything a year or more in the future. In the 1970s, utilities started using econometric and mixed end-use econometric models to produce long-term forecasts. Out went twelve-inch rulers, a previously powerful tool, as OPEC, inflation, industrial productivity, and energy efficiency entered our vocabulary and were directly addressed by the new methodology.
This econometric forecasting paradigm has since enjoyed a forty-year run as the predominant long-term load forecasting approach. But, as any well-trained econometrician will tell you, the underlying causal modeling structure leads to spectacular failures when its foundation changes.